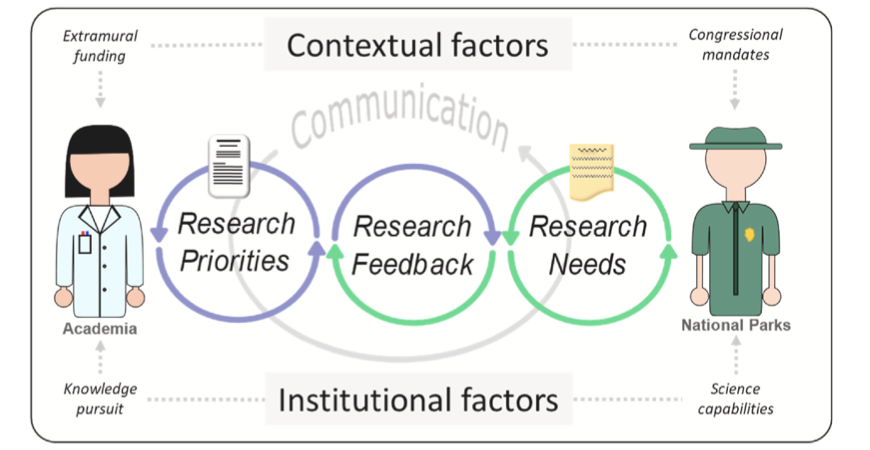
Recent UCM doctoral graduate Dr. Felber Arroyave and MCS professors Jenkins and Petersen published two papers exploring the impact of science policy on knowledge management and other systems-level dimensions of the US National Park System by applying methods of machine learning, network analytics, and management science – read more below.
1) Research alignment in the U.S. National Park System: Impact of transformative science policy on the supply and demand for scientific knowledge for protected area management (Journal of Environmental Management, 2024). * In collaboration with UCM's National Parks Institute director Steve Shackelton, and Yosemite and Sequoia National Park field stations director Breezy Jackson.
Abstract: The US National Park System encompasses diverse environmental and tourism management regimes, together governed by the 1916 Organic Act and its dual mandate of conservation and provision of public enjoyment. However, with the introduction of transformative science policy in the 2000's, the mission scope has since expanded to promote overarching science-based objectives. Yet despite this paradigm shift instituting “science for parks, parks for science”, there is scant research exploring the impact of the National Park Science Policy on the provision of knowledge. We address this gap by developing a spatiotemporal framework for evaluating research alignment, here operationalized via quantifiable measures of supply and demand for scientific knowledge. Specifically, we apply a machine learning algorithm (Latent Dirichlet analysis) to a comprehensive park-specific text corpus (combining official needs statements -i.e. demand- and scientific research metadata -i.e. supply-) to define a joint topic space, which thereby facilitates quantifying the direction and degree of alignment at multiple levels. Results indicate an overall robust degree of research alignment, with misaligned topics tending to be over-researched (as opposed to over-demanded), which may be favorable to many parks, but is inefficient from the park system perspective. Results further indicate that the transformative science policy exacerbated the misalignment in mandated research domains. In light of these results, we argue for improved decision support mechanisms to achieve more timely alignment of research efforts towards distinctive park needs, thereby fostering convergent knowledge co-production and leveraging the full value of National Parks as living laboratories.
2) Network embedding for understanding the National Park System through the lenses of news media, scientific communication and biogeography (Annals of the American Association of Geographers, 2024).
Abstract: The U.S. National Parks encompass a variety of biophysical and historical resources important for national cultural heritage. Yet how these resources are socially constructed often depends on the beholder. Parks tend to be conceptualized according to their (fixed) geographic context, so our understanding of this system of systems is dominated by this geographic lens. To expose the systemic structure that exists beyond their geographic embedding, we analyze three representations of the National Park System using park–park similarity networks according to their cooccurrence in (1) about 423,000 news media articles; (2) about 11,000 research publications; and (3) about 60,000 species inhabiting parks. We quantify structural variation between network representations by leveraging similarity measures at different scales: park level (park–park correlations) and system level (network communities’ consistency). Because parks are governed and experienced at multiple scales, cross-network comparison informs how management should account for the varying objectives and constraints that dominate at each scale. Our results identify an interesting paradox: Whereas park-level correlations depend strongly on the representative lens, the network communities are remarkably robust and consistent with the underlying geographic embedding. Our data-driven methodology is generalizable to other geographically embedded socioenvironmental systems and supports the holistic analysis of systems-level structure that might elude other approaches.